

Embeddings also achieved 99.85% accuracy on data source classification through k-means clustering. Powered by OpenAI’s embeddings of these astronomical reports, researchers are now able to search for events like “crab pulsar bursts” across multiple databases and publications. JetBrains Research’s Astroparticle Physics Lab analyzes data like The Astronomer’s Telegram and NASA’s GCN Circulars, which are reports that contain astronomical events that can’t be parsed by traditional algorithms. Linear probe classification over 7 datasetsĮxamples of the Embeddings API in Action JetBrains Research In machine learning literature, when using a linear classifier, this classification task is called a “linear probe.” Our text similarity models achieve new state-of-the-art results on linear probe classification in SentEval ( Conneau et al., 2018), a commonly used benchmark for evaluating embedding quality. One popular use of embeddings is to use them as features in machine learning tasks, such as classification. Similarity_score = np.dot(embedding_a, embedding_b) import openai, numpy as npĮmbedding_a = respĮmbedding_b = resp In most applications, the embeddings can be pre-computed, and then the dot product comparison is extremely fast to carry out. The result is a “similarity score”, sometimes called “ cosine similarity,” between –1 and 1, where a higher number means more similarity. To compare the similarity of two pieces of text, you simply use the dot product on the text embeddings.
#Vectorize font how to
The code for how to visualize embedding space in 3D dimension is available here. To visualize the embedding space, we reduced the embedding dimensionality from 2048 to 3 using PCA.

The different categories show up as 5 clear clusters in the embedding space. We randomly selected 100 samples from the dataset covering 5 categories, and computed the embeddings via the /embeddings endpoint. The following interactive visualization shows embeddings of text samples from the DBpedia dataset:Įmbeddings from the text-similarity-babbage-001 model, applied to the DBpedia dataset. These models are useful for many tasks including clustering, data visualization, and classification. Text similarity models provide embeddings that capture the semantic similarity of pieces of text. Text similarity : Captures semantic similarity between pieces of text.
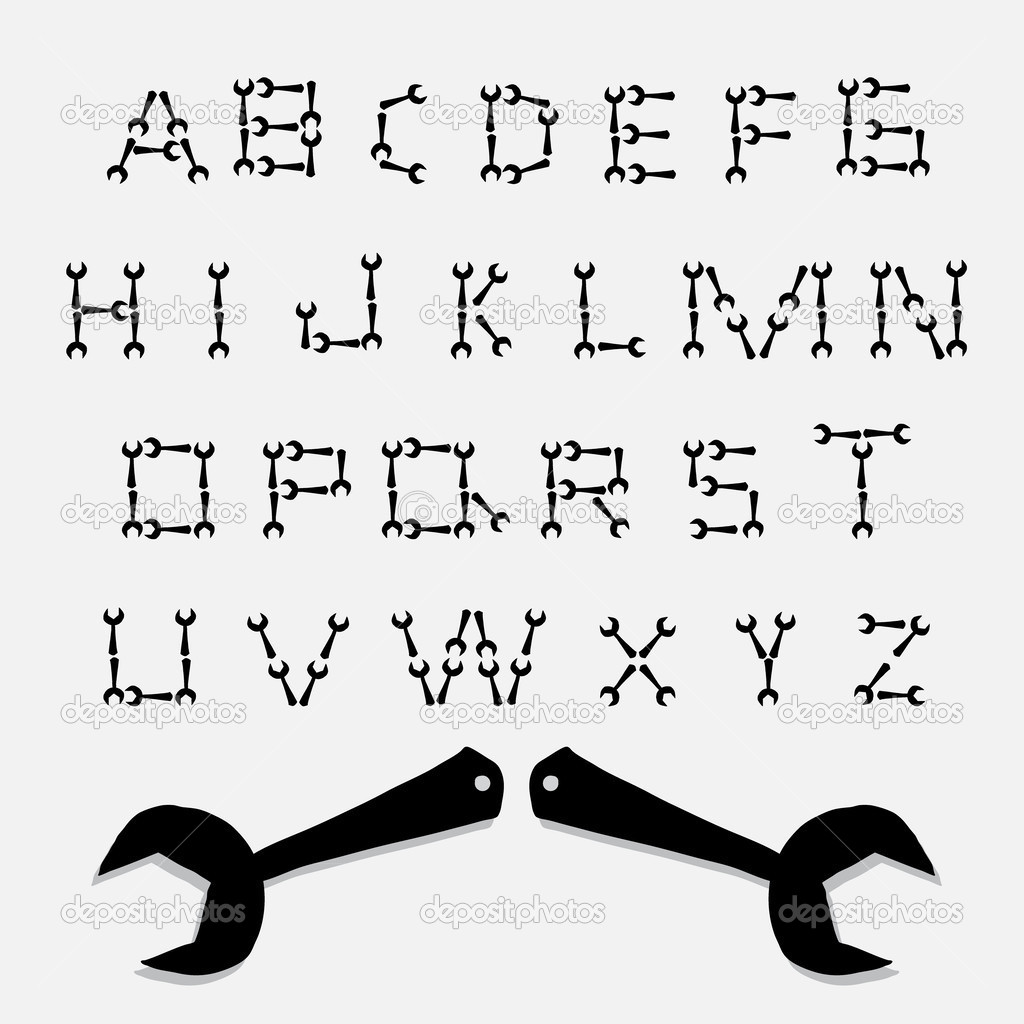
The models take either text or code as input and return an embedding vector. We’re releasing three families of embedding models, each tuned to perform well on different functionalities: text similarity, text search, and code search.
